KONFERENZPROGRAMM
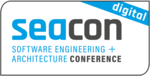
Reinforcement learning - the future of robot programming !?!
Reinforcement Learning (RL) is a type of machine learning technique that enables to learn from experiences and interactions between intelligent agents and the environment. This ability is not naturally provided by classical supervised learning wherein the entire training process is based on past experiences. In RL, the training target is mainly formulated as composite rewards for the decisions made by agents at particular states (of the system in the problem environment). Over time, the agents learn to improve their future cumulative rewards which, depending on the problem being solved, can appear to address a particular case where a specific goal is achieved or an optimal state is reached. This phenomenon could help addressing the problems for which insufficient data or modeling knowledge is available and also problems with significant levels of uncertainly. In particular, such cases are referred to as partially observable problems and regarding the fact that most RL solutions are provided under the assumption of the Markov property, RL methods nowadays are most significantly incorporated to address Partially Observable Markov Decision Processes (POMDP). RL has recently had a significant contribution to robotic controllers at different industrial roles. For the lowest level, training robots how to grasp an object quickly with a correct amount of force and appropriate rotations of the hand-like end-effectors as well as the paths the serially connected prismatic arms should move through can be learned via the use of RL. For curious people in the field, Deep-mind has provided efficient simulation environments and diverse parametric functionality to facilitate an enhanced understanding of the problem space. In addition, RL can assist with even more complex situations, such as when there is a variety of sensory data, but insufficient knowledge about the environmental reaction to the decisions made by the robot. The RL-controller can learn how to improve its reward or reach its goal optimally by choosing appropriate actions and receiving the corresponding feedback from the environment. Siemens Corporate Technology is one of the many who used RL based robotic controllers to drive its manufacturing assembly processes. In general, in a real-world application of RL for robotic control, the process of building the RL controller begins with defining an appropriate reward, identifying appropriate state variables and listing action space. Selecting an appropriate RL method out of value based, policy based or mixed approaches such as actor-critic methods is the next step. Simulations are repeated in an episodical manner and the success of the developed controller in achieving the learning objectives can be monitored in the growth (or decline) of the value of the reward. RL has recently played a more significant role in providing decentralized and interconnected autonomous systems where multiple agents negotiate and cooperate to handle complex tasks and multi-objective problems. Such solutions are referred to as Multi-agent Reinforcement Learning (MARL). Depending on the problem, such environments are massively complex for humans to model and an appropriate solution cannot be achieved without dealing with the curse of dimensionality without the use of MARL techniques. Distributed manufacturing systems are the next generation of such application as a significant number of industrial and academic research projects have recently been undertaken on the topic.
Dr. Fouad Bahrpeyma (IEEE Member) is a postdoctoral researcher at HTW Dresden, with the Smart Production Systems group. He received his PhD in Artificial Intelligence from Dublin City University, Insight Center for Data Analytics in 2020. He also has a M.Sc. degree in AI and a B.Sc. degree in computer science. He is co-supervising PhD, M.Sc. and undergraduate students, and is a co-author/author of various journal papers, conference papers and chapter-books in the field of Artificial Intelligence.
His areas of research are mainly Self-organizing systems, Multi-Agent Reinforcement Learning, Deep Learning, Smart Manufacturing Systems, Cognitive science, Signal processing and Time Series Analysis. He has an ongoing research focus on Multi-agent reinforcement learning for decentralized management approaches and distributed robotic controllers in Smart Manufacturing Systems, along with Brain-Computer Interfaces (BCIs) for developing brain controlled robotic systems. He is involved in various R&D projects such as CASO and Arrowhead tools.